From Data Silos to Adaptive Reactions: Using RAG to Ensure Reliable GenAI
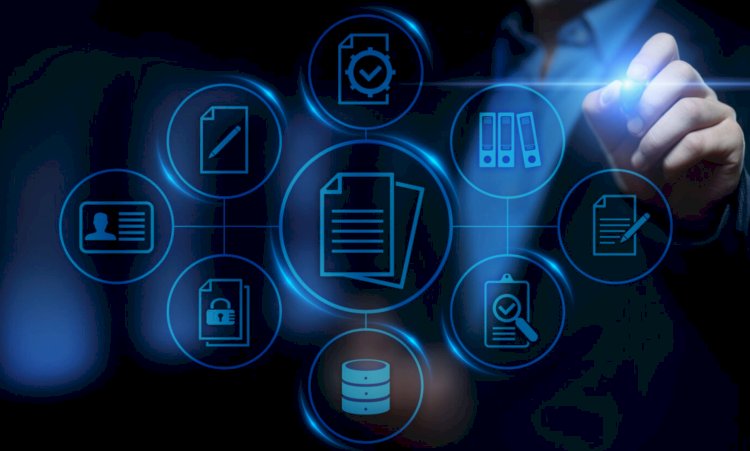
The possibilities in the field of GenAI are endless. The LLMs have gone from creating rhymes to writing code. The matter at hand is not whether GenAI can provide precise and reliable information, but rather whether we can trust it to do so. Retrieval-Augmented Generation (RAG) is applied at this point.
The Achilles’ Heel of Traditional LLMs
While the new generation of large language models (LLMs) excels at generating coherent narratives, their knowledge base is inherently limited and static. These models lack awareness of topics beyond their training data, leading to frequent errors and outdated responses. Additionally, complex queries often result in incoherent answers.
Retrieval Augmented Generation (RAG): A Solution
Retrieval augmented generation (RAG) serves as a bridge, integrating pure generation with robust factual support. It functions like a skilled research assistant specifically designed for LLMs. RAG leverages a vast external knowledge base, encompassing documents, databases, and websites, to enhance responses. Using advanced information retrieval techniques, RAG identifies the most relevant snippets from this knowledge base. An LLM equipped with RAG can produce responses that are not only creative but also factually accurate.
Practical Implications of RAG
The practical applications of RAG extend well beyond theoretical discussions. For instance, sophisticated chatbots benefit significantly from RAG. Traditional customer service chatbots often struggle with complex issues, but RAG-powered bots can reference product manuals, procedures, FAQs, and even real-time data to provide precise and personalized responses. This capability transforms customer service, enabling chatbots to address intricate problems effectively.