AI Security: Safeguarding the Future of Artificial Intelligence
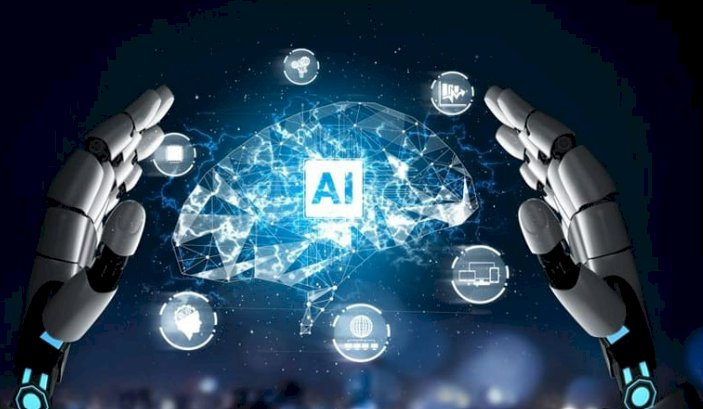
As artificial intelligence (AI) continues to revolutionize industries and transform our daily lives, the importance of AI security has become increasingly critical. Ensuring the security of AI systems is essential for preventing misuse, protecting data, and maintaining public trust. This article explores the key challenges and strategies in AI security, highlighting the need for a comprehensive approach to safeguarding the future of AI.
Understanding AI Security
AI security involves protecting AI systems from threats and vulnerabilities that could compromise their functionality, data integrity, or user privacy. This encompasses a wide range of concerns, including:
1. Data security: Protecting the data used to train and operate AI systems from unauthorized access and tampering.
2. Algorithm Integrity: ensuring that AI algorithms function as intended and are not manipulated or corrupted.
3. Privacy Protection: Safeguarding the privacy of individuals whose data is used or processed by AI systems.
4. Robustness and Resilience: Building AI systems that can withstand and recover from attacks and failures.
Key Challenges in AI Security
1. Data Poisoning: Adversaries can manipulate training data to corrupt the AI model, leading to incorrect or harmful outputs. Ensuring the integrity and quality of training data is crucial.
2. Model Inversion Attacks: Attackers can reverse-engineer AI models to extract sensitive information about the training data, potentially compromising privacy.
3. Adversarial Attacks: Malicious actors can create inputs specifically designed to deceive AI systems, causing them to make erroneous decisions. These attacks can be difficult to detect and mitigate.
4. Black Box Nature of AI: Many AI systems, especially deep learning models, operate as "black boxes" with limited transparency. This lack of interpretability can make it challenging to identify and address security vulnerabilities.
5. Algorithmic Bias: AI systems can inadvertently learn and perpetuate biases present in training data, leading to unfair and discriminatory outcomes. Ensuring fairness and accountability is a significant concern.
Strategies for Enhancing AI Security
Addressing the challenges of AI security requires a multi-faceted approach. Here are some key strategies:
1. Secure Data Management: Implement robust data governance practices to ensure the integrity, confidentiality, and availability of data. This includes encryption, access controls, and regular audits.
2. Adversarial Training: Train AI models using adversarial examples to improve their resilience against adversarial attacks. This involves exposing the model to a variety of malicious inputs during training.
3. Explainability and Transparency: Develop AI models that provide clear explanations for their decisions. Transparent AI systems can help identify and mitigate security risks more effectively.
4. Regular Security Audits: Conduct regular security assessments and penetration testing to identify vulnerabilities in AI systems. This proactive approach helps address potential threats before they can be exploited.
5. Bias Mitigation Techniques: Implement techniques to detect and reduce bias in AI models. This includes diverse and representative training data, fairness constraints, and continuous monitoring.
6. Collaboration and Standards: Collaborate with industry partners, researchers, and regulators to establish best practices and standards for AI security. A unified approach can enhance the overall security posture of AI technologies.
The Role of Policy and Regulation
Government policies and regulations play a vital role in promoting AI security. Policymakers need to balance innovation with security and privacy concerns. Key areas of focus include:
1. Data Protection Laws: Enforcing stringent data protection regulations to safeguard the privacy and security of personal information used by AI systems.
2. Ethical Guidelines: Establishing ethical guidelines for AI development and deployment to ensure responsible and secure use of AI technologies.
3. Security Standards: Developing and enforcing security standards specific to AI systems, including guidelines for secure development, deployment, and maintenance.
Conclusion
AI security is a critical component in the responsible development and deployment of artificial intelligence. By addressing the unique challenges and implementing comprehensive security strategies, we can protect AI systems from threats and ensure their safe and ethical use. As AI continues to evolve, ongoing collaboration between industry, academia, and government will be essential in safeguarding the future of AI and maintaining public trust in these transformative technologies.